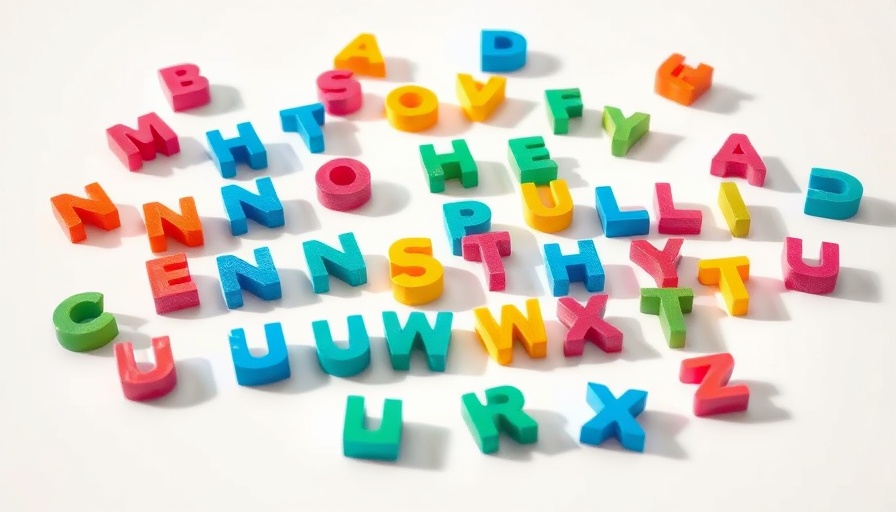
A New Era of Language Learning
The BabyLM Challenge has emerged as a groundbreaking initiative aimed at refining the way artificial intelligence (AI) learns language. Traditionally, language models (LMs) rely on colossal datasets, often consisting of over a trillion words, far exceeding the cumulative language exposure of an average 13-year-old. But what if we could harness the efficient learning strategies of infants?
Human Learning vs. Machine Processing
Children acquire language through exposure to roughly 10 to 100 million words by their early teenage years, significantly less than what AI typically consumes. This disparity raises a critical question: Are we misguiding our approaches to teaching machines language? If LMs continue to grow in size and data consumption, researchers predict a looming shortage of new data by 2028, signaling an urgent need for more sustainable training methods.
The BabyLM Challenge Explained
Launched to tackle these inefficiencies, the BabyLM Challenge asks participants to develop models adept at learning from smaller datasets. With tracks featuring 10 million and 100 million words, this competition seeks solutions that mimic the human experience more closely. The challenge encourages creativity by allowing researchers to utilize any available model and processing method, resulting in a variety of innovative approaches.
Insights from the Challenge
Since its inception in 2023, the BabyLM Challenge has shown promising results in enhancing language models through human-inspired learning strategies. By focusing on techniques that reflect how infants learn—such as beginning with simple texts and gradually progressing to complex ones—participants have found pathways toward productive innovation. Some have also explored recombining data in novel ways to mitigate the limitations of smaller datasets while seeking to unify vision and language in their models.
Inspiration from Psychology
Innovation often stems from necessity. The limitations faced by academic researchers, who frequently lack the substantial funding of major tech companies, have become a powerful motivator. Their constraints push them to explore beyond the traditional boundaries of language models, drawing on interdisciplinary knowledge, particularly from developmental psychology. This fusion of fields enriches the understanding of language acquisition and creates a fertile ground for new ideas in AI.
Environmental Considerations and Future Outlook
As the challenge unfolds, its implications for the environment cannot be overlooked. The energy-intensive nature of large language model training is under scrutiny, urging researchers and designers to consider the ecological footprint of their innovations. Greater awareness of this issue has prompted a push towards efficiency, advocating for the development of intelligent systems that require less power and data without sacrificing performance.
A Community of Innovators
Participants in the BabyLM Challenge have fostered a vibrant community, sharing insights, challenges, and breakthroughs. This collaborative space encourages experimentation, allowing researchers to test new hypotheses and ultimately evolve the field of language modeling. It's a reminder that science is not merely about competition but also about community and mutual advancement.
Moving Forward: The Next BabyLM Challenge
Set for November 2025, the next iteration of the BabyLM Challenge promises to further explore these avenues. As researchers prepare their submissions, the anticipation builds not just for the potential discoveries that will arise, but for the valuable discourse that will accompany them. The dialogue around LM efficiency will continue and likely influence not only how we design AI but also how we understand language acquisition itself.
In a world increasingly reliant on AI, the BabyLM Challenge serves as a vital reminder of the profound lessons we can learn from our youngest members of society. Adopting methods that mirror childhood language development strategies may not only enhance machine learning but also foster a deeper understanding of language and communication among humans.
Write A Comment